Article
Taking Media QC to a new level with AI & MI – A Primer
Delivering stellar media experiences is what sets the best offerings apart, and Media QC is playing a pivotal role in this. Using Artificial Intelligence and Machine Learning within Media QC solutions is fast emerging as a game-changer.
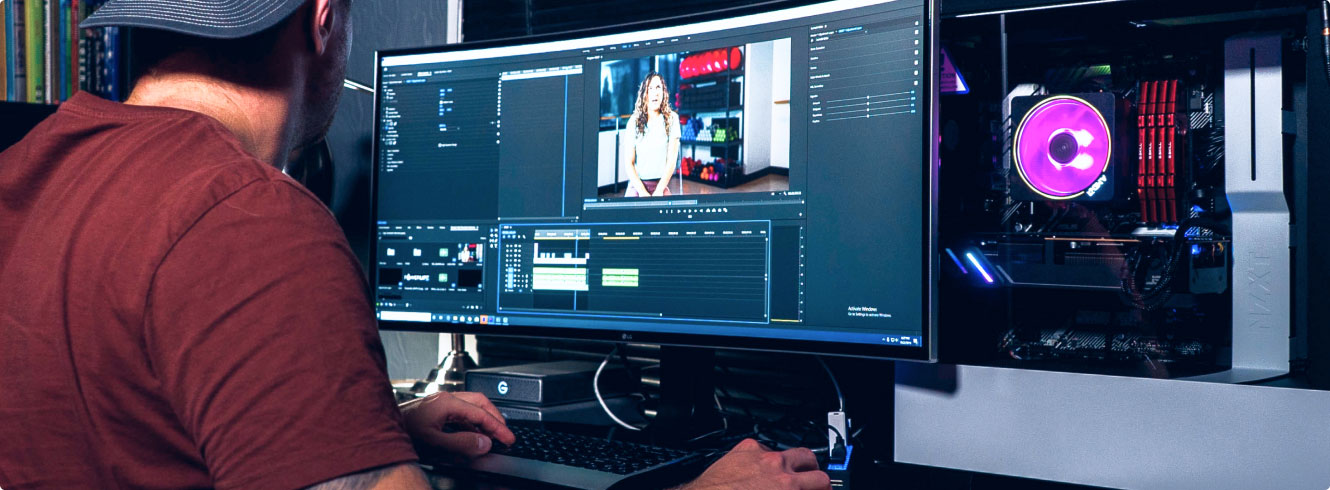
The media industry keeps adapting to the latest technological trends and is becoming more “viewer-friendly.” Instead of broadcasting linear media (i.e., media that has to be viewed sequentially, from beginning to end), the media industry is shifting to non-linear media. They give more control to viewers by offering them the choice to interact with the media available to them.
In 2019, the total number of OTT and digital streaming viewers crossed the 235 million mark in the US – that accounts for 71.2% of the country’s population (eMarketer). Along with this, CBS also expects a 50% boost in non-linear viewing of television content. With the increase in digital media popularity, broadcasters now have to deliver a lot of content to a broader range of audiences and screens. In turn, giving rise to several pre, mid, and post-production issues, such as video/audio quality, workflow issues, etc.
This drastic shift from linear to non-linear media has made it crucial for broadcasters to focus primarily on media QC.
What is media QC?
Media quality control is the process of ensuring that a media file meets the specifications and standards required for the target audience. From video/audio file formats to content regulation guidelines, the media QC process verifies everything before it is submitted for approval.
With streamlined media QC processes, broadcasters have:
- Improved workflows
- Optimized content delivery to a wide range of audiences
- Enhanced video/audio quality
- Ensured the file meets required content guidelines
- Increased media approval rate
How media QC is evolving
Media QC has come a long way. In its early stages, media QC automation was limited to specific tasks, such as checking frame rate, content structure, resolution, etc. Jump to 2021; the media industry has leveraged technology that helps broadcasters find perceptual errors using different techniques, such as visual text recognition, language detection, interlace artifacts, defective pixels, etc.
With AI/ML reaching new heights in object detection, content classification, etc., media QC’s scope expanded. Now, AI/ML techniques are being used in multiple areas, including content moderation, filtering, indexing, etc. We take a look into this in detail below.
Role of AI/ML in transforming media QC capabilities
From quality to compliance, media QC covers it all. AI/ML takes media QC to another level and ensure error-free quality checks, reduced turnaround time, and reduced operational costs. AI/ML helps in:
Delivering a personalized viewer experience
A customized user experience is a key to ensuring retention and loyalty. Personalizing user experiences manually on digital streaming apps or OTT platforms was very time-consuming. By integrating AI/ML techniques, the media industry reduced the time spent on optimizing experiences and ensured an accurate analysis of large amounts of viewer data.
With the combination of big data analysis, AI/ML techniques help the media industry to deliver personalized viewer experiences. Most of these techniques analyze viewer information, watch history, search history, and preferences.
For example, Netflix leveraged AI and ML techniques to give recommendations that best meet a specific viewer’s interest. Their algorithm is based on previously searched and viewed videos. Although complex, Netflix has come out to streamline the process of offering a personalized viewer experience.
Improving content searches
Another important factor in delivering exceptional viewer experiences is accurate, fast search capabilities. When OTT platforms come into the picture, the content was usually classified based on genre, language, actors, year of release, etc. These parameters were easily identified and the content classification process was accurate. But, with the increase in content volume, it became difficult to track and classify content.
In recent years, AI/ML techniques have also been introduced in this process. It not only helps optimize and automate the entire process, but also classifies content beyond the usual attributes. Image recognition is one of the most powerful applications of AI/ML in improving content searches. With the help of the metadata produced through recognizing images, it optimizes the process of clustering content based on context, search, or selection.
Fast-tracking content compliance
The rules of regulations that media content must adhere to can vary from region to region. Traditionally, this process was carried out manually by filtering content for regulatory compliance. They followed a review workflow – i.e., the content underwent multiple rounds of review, and if failed at any stage, it was sent back to the editing phase. This process was time-consuming, expensive, and inaccurate.
In recent years, AI/ML has proven to be the solution to these problems. Meeting global content moderation standards with 100% accuracy seemed possible only through automation. By automating the process, broadcasters eliminated several hurdles in the QC process, enhanced the content review speed, and improved the review accuracy.
With the combined technology, it gets easier to perform in-depth checks in content, such as identifying brand names, violence, explicit content, alcohol, smoking, religious symbols, etc. Although the automated process overcomes many QC issues and helps evolve the entire process, a human touch is still required to confirm the validity of patterns and assist machines in refining the end result.
Eliminating lip-sync issues
Video/audio quality is key to meeting viewer expectations. The most common issue many broadcasters faced is synchronization between audio and video. Manually editing video and audio files to keep them in sync was time-consuming and required a lot of effort.
Broadcasters leveraged image processing, facial tracking, facial recognition, lip activity detection, speech identification, and neural networks to automate the audio-video sync error detection process. It is a three-step process. The first step is to extract faces and track lip movement in a video. Next, the audio files are extracted and analyzed. Finally, the extracted video and audio files are matched.
With such a detailed process, ML offers a more accurate and faster approach to detecting lag and audio issues in every frame of a media file. This, in turn, eliminates the lip-sync issues and helps broadcasters deliver high-quality content to viewers.
Refining media captions and subtitles
Along with synchronization issues, another major problem broadcasters face is inaccurate captions and subtitles. As broadcasters started catering to a global audience, with streaming and OTT platforms, manually going through hours of audio/video content to generate subtitles showed inaccurate results.
With AI/ML in the mix, broadcasters can now accurately generate captions and subtitles in half the amount of time. Machine learning also helps check the correctness of captions used in a video file and the alignment between the audio and captions. Automatic speech recognition has seen an 85% accuracy rate via ML.
Although these techniques help solve a lot of issues, a few problems, such as handling variable accents, multiple people speaking simultaneously, etc., still require manual processes. Combining the cutting-edge AI/ ML techniques with a manual review process, broadcasters cut costs, generate accurate captions, and reduce turnaround time.
Endnote
With the drastic shift in the media industry, media QC is the key to delivering high-quality video experiences. Leveraging new technologies, such as artificial intelligence, machine learning, deep learning, natural language processing, and neural networks, is bound to take media QC to a whole new level. We can already see the change in OTT platforms, such as Netflix or Hulu, that have optimized user experiences with the help of AI. We are bound to see more technological innovations being used in delivering high-quality content to viewers.